AI Activation in Private Equity: What Success Looks Like
Written by
Jacob Zweig, Managing Director
Published
September 12, 2024
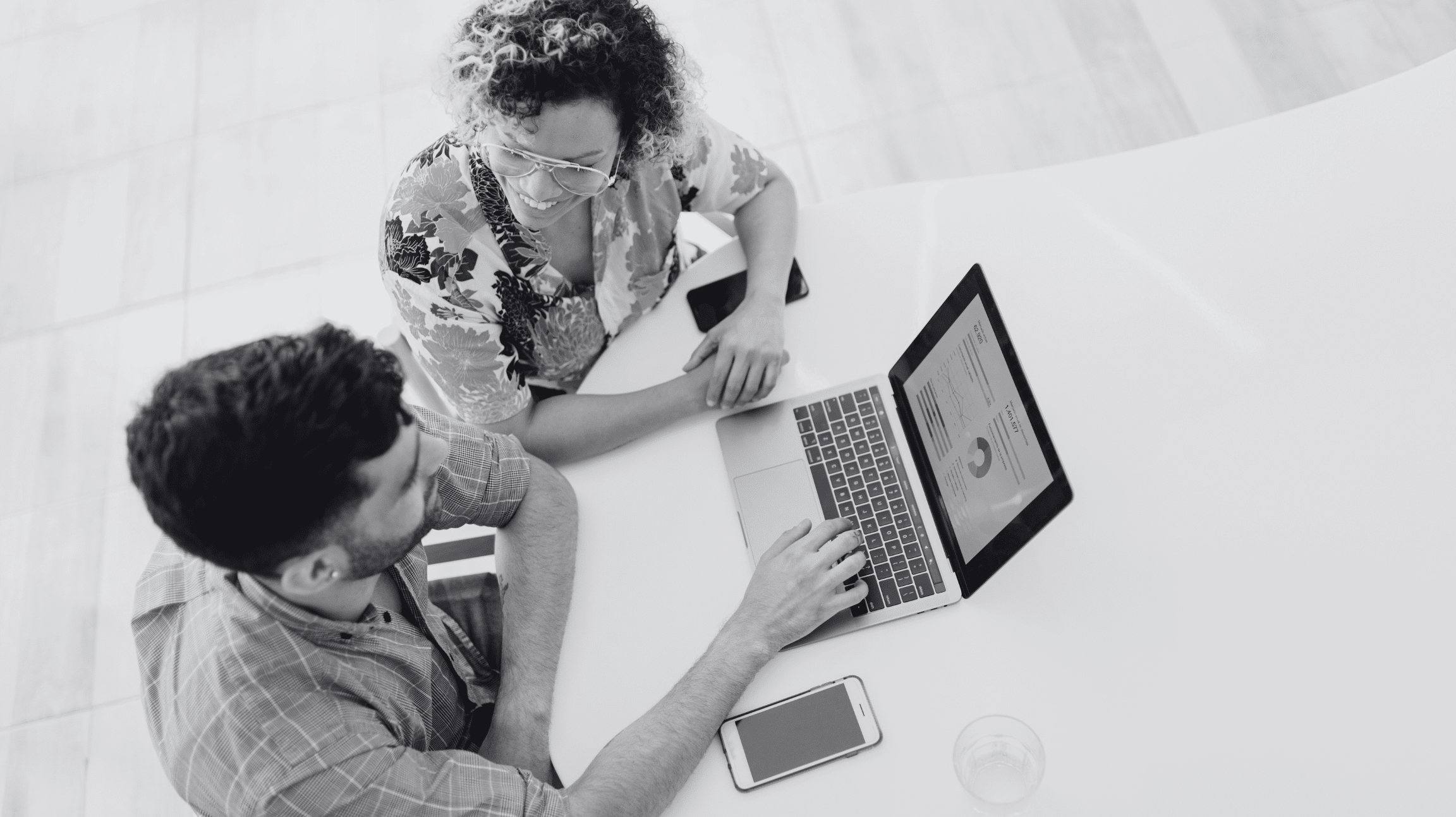
For private equity firms, artificial intelligence (AI) stands out as a game-changer, offering unprecedented opportunities for enhancing decision-making, optimizing operations, and driving value creation. AI success in a private equity firm involves a strategic approach that encompasses advanced analytics, robust AI operations, and impactful AI applications. Here’s a detailed look at what AI success looks like in this context.
Advanced Analytics and AI Fundamentals
Clearly Defined AI Strategy
Successful firms identify and define AI use cases that align with their business objectives and have the potential for significant impact. These use cases guide AI investments and development efforts.
Implementation of Predictive Models and ML Algorithms
AI success begins with the effective implementation of predictive models and machine learning (ML) algorithms in production environments. These tools can analyze vast amounts of data to predict future trends, identify investment opportunities, and mitigate risks.
In-House Data Science Expertise
Building a strong in-house team of data scientists, statisticians, and ML engineers is crucial. These experts drive AI initiatives, develop sophisticated models, and ensure the effective use of AI across the firm.
Experimentation with Advanced ML Techniques
Experimenting with and implementing advanced machine learning techniques, such as deep learning, enables the firm to tackle complex problems and discover new insights.
Maintaining a Feature Store
A feature store, which centralizes and standardizes features used in various models, is essential for efficient and consistent AI model development.
AI Operations and Infrastructure
To fully realize the benefits of AI, it’s essential to have robust operations and infrastructure in place.
Formal MLOps Framework
Adopting a formal MLOps framework is critical for managing the lifecycle of AI models, from development to deployment and monitoring. This framework ensures reliability, scalability, and continuous improvement of AI systems.
Monitoring Dashboards
Reliable monitoring dashboards are essential for tracking the health and performance of AI models in production. These tools provide real-time insights and enable proactive maintenance.
Integration with Operational Systems
Successful AI implementation extends beyond research projects, integrating AI models with operational systems, workflows, or core products to drive tangible business value.
Access to Data and Compute Resources
Providing data scientists with full access to necessary data sources and compute resources is crucial for maximizing AI model performance and innovation.
Versioning and Tracking
A robust process for versioning and tracking changes in AI models and datasets ensures transparency, reproducibility, and continuous improvement.
AI Applications and Use Cases
AI applications can provide substantial improvements in various business processes. Focus on practical use cases that drive operational efficiency and enhance customer experiences.
Computer Vision Applications
Implementing computer vision technologies, such as image recognition, object detection, and object tracking, can significantly enhance business processes and operational efficiency.
Natural Language Processing (NLP)
Utilizing NLP technologies for tasks like text classification, sentiment analysis, and chatbots enhances communication, customer service, and data analysis capabilities.
Large Language Models (LLMs)
Exploring and implementing large language models (LLMs) for various business applications can unlock new opportunities for automation and intelligence.
Supply Chain Optimization
AI-driven demand forecasting, inventory optimization, and other supply chain-related tasks enhance efficiency, reduce costs, and improve service levels.
AI-Driven Recommendation Systems
Implementing AI-driven recommendation systems enhances personalization and customer experience, driving higher engagement and conversion rates.