Preparing Your Data for AI: 5 Key Requirements
Written by
Ajit Monteiro, CTO & Co-Founder
Published
January 2, 2024
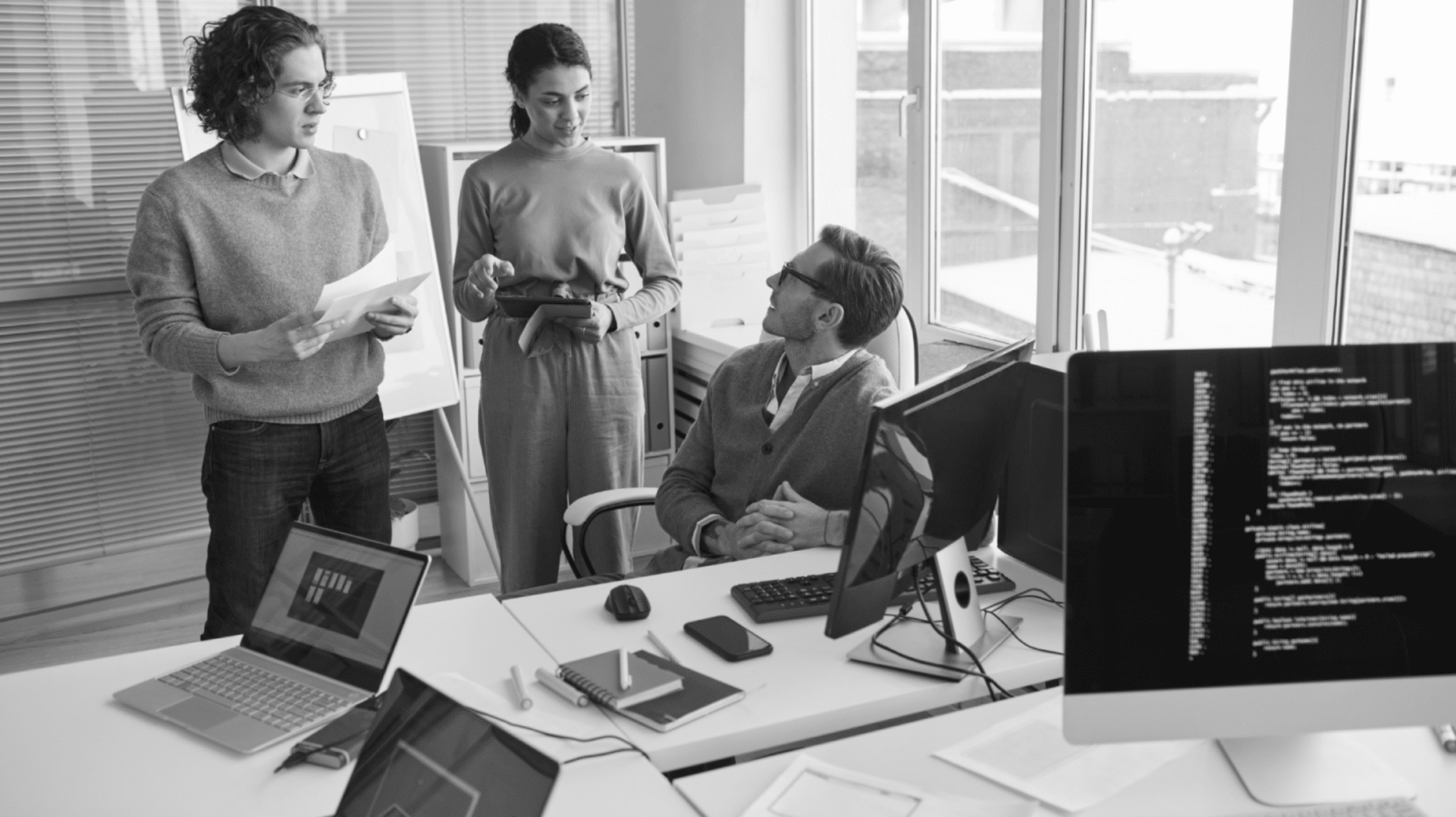
Data serves as the backbone of AI, with its availability and quality serving as foundational pillars for successful integration. Evaluating AI readiness entails a thorough assessment of the existing data landscape within the organization. This involves identifying pertinent datasets, evaluating their quality, and ensuring alignment with predefined AI use cases and objectives.
Based on our work in helping our clients unlock the power of their data, we’ve identified five key data elements that are absolutely crucial for laying the groundwork for AI. These elements aren’t just about getting your data ready; they’re about putting you in the best possible position to really get value out of AI for your business.
1. You must have a modern data strategy
Aware:
Siloed teams and applications; Little to no standards for data management
Reactive:
Data organized into a consolidated data platform but lacks standardization; sharing data is across a few teams based on needs
Proactive:
Broader push of automated, accurate and usable data insights to all levels of the organization
Managed:
Data insights, advanced analytics and machine learning are widely used and iterated based on real world results
Optimized:
Data as a significant competitive advantage; Scaling of various data products; Modern Data Org values embedded into operational culture
2. Your data must be in the cloud
The cloud has become the preferred home for data in the era of AI. Cloud-based data storage offers unparalleled advantages in terms of scalability, accessibility, and cost-effectiveness. By leveraging cloud platforms, organizations can seamlessly integrate AI applications into their workflows without moving large amounts of data to the AI algorithms. It also allows AI workloads to be run on an ad-hoc basis, enabling organizations to pay for infrastructure only when needed. This cloud-native approach not only facilitates efficient data processing but also provides the flexibility to scale resources according to the demands of AI workloads.
3. Your data must be integrated and automated
For AI to thrive, data integration and automation are non-negotiable. Siloed data can be a significant impediment to AI effectiveness. Integration ensures that data flows seamlessly across your organization, breaking down data silos and creating a unified view. Automation streamlines the data pipeline, reducing manual efforts and enhancing the speed at which data becomes available for AI processes.
4. Your data must be unified
Unifying data involves creating a singular, coherent view of your entire data landscape. This encompasses diverse data sources, formats, and structures. A unified data approach ensures that AI models have access to a holistic dataset, enabling more accurate insights and predictions. Data unification also simplifies the process of data governance, as there is a single source of truth for all data-related activities.
5. Your data quality must be a constant focus
The quality of your data directly correlates with the success of your AI initiatives. Poor-quality data can lead to inaccurate predictions, flawed insights, and compromised AI performance. Impeccable data quality involves thorough cleansing, validation, and maintenance processes. Regular audits and checks should be in place to ensure that your data remains accurate, consistent, and reliable over time.
How to Create Business
Value with AI
Laying a solid foundation for AI begins with ensuring your data is not just available but optimized for AI applications. A modern data strategy, cloud-based storage, integration and automation, unified data, and impeccable data quality collectively form the pillars of AI readiness. By incorporating these must-haves into your data practices, you pave the way for successful AI implementation, unlocking the full potential of AI in your organization.
To help organizations maximize the value of AI, we’ve developed tailored, industry-specific guides for Banking, Private Equity, and Software.
Get Started
OneSix helps companies build the strategy, technology, and teams they need to unlock the power of their data.