Laying the Groundwork for AI: Establishing a Data-Driven Culture
Written by
Mike Galvin, Managing Director
Published
July 11, 2024
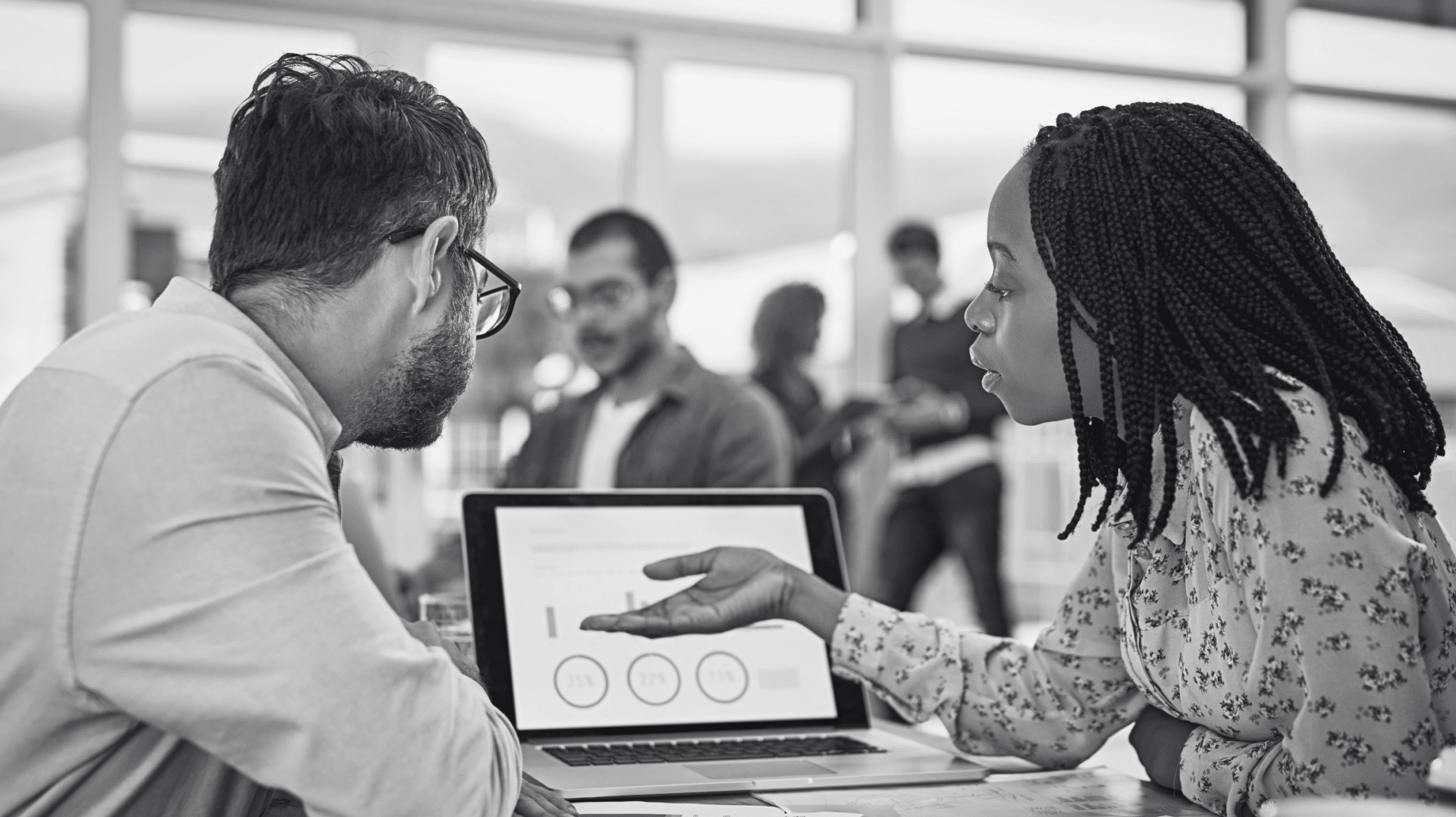
A recent Deloitte survey revealed that 51% of CEOs consider data challenges the primary obstacle to generating business value with Artificial Intelligence (AI), highlighting the critical importance of data readiness in AI implementation.
AI’s power lies in its ability to analyze vast amounts of data and derive actionable insights. However, this process hinges on the quality and integration of the data fed into AI systems. Poor data quality, fragmented data sources, and a lack of integration between systems can significantly hamper the effectiveness of AI initiatives. Let’s delve into these challenges and explore how building a data-driven culture can serve as a solution.
1. Poor Data Quality: AI systems rely on high-quality data to function effectively. Data quality issues, such as inaccuracies, inconsistencies, and missing values, can lead to erroneous insights and decisions. Poor data quality can stem from various sources, including human error, outdated information, and inadequate data governance practices.
2. Fragmented Data Sources: In many organizations, data is scattered across multiple systems, departments, and formats. This fragmentation creates silos that hinder the comprehensive analysis needed for AI to be effective. Fragmented data sources result in a lack of a unified view, making it difficult to draw meaningful conclusions.
3. Lack of Integration Between Systems: Integration issues arise when disparate systems and technologies cannot seamlessly communicate and share data. This lack of integration prevents the smooth flow of information, leading to inefficiencies and missed opportunities. Incompatible data formats, legacy systems, and insufficient interoperability are common culprits.
In a recent Deep Dish Data panel, best-selling author Joe Reis shares:
How often do you find that data is ready for any particular use case or consumption? Most datasets are really gnarly. We need to focus on establishing the foundation and getting the fundamentals in place.
Joe Reis
Laying the Foundation for a Data-Driven Culture
Creating a data-driven culture involves more than just deploying advanced technologies; it requires a holistic approach to managing data effectively. By focusing on the key areas below, organizations can transform their data into a valuable asset that drives informed decision-making and competitive advantage.
1. Establishing Data Governance
Effective data governance is the cornerstone of high-quality data. Implementing robust data governance policies ensures data accuracy, consistency, and reliability. This involves defining data standards, establishing data ownership, and implementing processes for data validation and cleansing.
2. Centralizing Data Management
To combat fragmented data sources, organizations should centralize data management. This can be achieved through data warehouses, data lakes, or cloud-based platforms that consolidate data from various sources. Centralized data management provides a unified view, enabling comprehensive analysis and facilitating better decision-making.
3. Enhancing Data Integration
Integrating data across systems requires the adoption of modern data integration tools and technologies. Application Programming Interfaces (APIs), middleware, and data integration platforms can facilitate seamless data exchange between systems. Emphasizing interoperability and adopting industry standards can further streamline integration efforts.
4. Promoting Data Literacy
Building a data-driven culture goes beyond technology and processes—it involves people. Promoting data literacy across the organization empowers employees to understand, interpret, and leverage data effectively. Training programs, workshops, and fostering a culture of continuous learning are key to enhancing data literacy.
5. Leveraging AI for Data Quality
AI itself can be harnessed to improve data quality. Joe Reis points out: “If the approaches to data management and governance that we’ve taken for the last several decades are having mixed results, will AI be a hail mary to make it work? I’m willing to take the bet that maybe this is worth considering.” Machine learning algorithms can detect anomalies, automate data cleansing, and validate data entries. By leveraging AI for data quality management, organizations can ensure that their data is accurate, consistent, and reliable.
Breaking Down Data Silos: A Success Story
How a Private Equity firm unified data for enhanced portfolio insights and reduced risk
Our client, a private equity firm managing a diverse portfolio of investments, faced challenges in leveraging data for strategic decision-making. The firm needed a modern data infrastructure to consolidate disparate datasets, enhance analytical capabilities, and gain deeper insights into overall portfolio performance.The implementation of a comprehensive data infrastructure provided the private equity firm with a holistic view of portfolio performance while ensuring data security and integrity. This empowered the firm to make more informed decisions, enhance operational efficiency, and gain deeper insights into their investments, ultimately mitigating risks and fostering long-term profitability.
Pro Tip: Start Small with Rule-Based Systems
As Winston Churchill famously said, “perfection is the enemy of progress.” While it’s important to focus on the above holistic approach to AI-ready data, you don’t necessarily need to wait until you reach the finish line before you can get started with AI. There’s a common misconception that you need enormous volumes of data to get started. But it’s not true. The reality is that you can start small and still make significant strides.
One approach is to begin with rule-based systems. These systems use predefined rules to process data and make decisions, providing immediate value without the need for massive datasets. Think of it as laying a solid foundation upon which you can build more sophisticated machine learning models over time. Starting with rule-based systems allows you to:
1. Gain Initial Insights: Even with limited data, rule-based systems can uncover valuable patterns and trends.
2. Improve Gradually: As you collect more data, you can refine and enhance your models, gradually transitioning to more advanced machine learning solutions.
3. Mitigate Risks: By starting small, you can test and validate your AI strategies without the risk of over-investing in unproven approaches.
The key to successful AI and ML outcomes isn’t the volume of your data—it’s how effectively you use the data you have.
Transforming Data into a Valuable Asset
Overcoming data quality and integration challenges is not merely a technical endeavor—it is a strategic initiative that transforms data into a valuable asset. By building a data-driven culture, organizations can unlock the full potential of their AI investments. High-quality, integrated data serves as the foundation for AI to deliver accurate insights, drive innovation, and create a competitive advantage.
By establishing robust data governance, centralizing data management, enhancing data integration, promoting data literacy, and leveraging AI for data quality, organizations can overcome these challenges. Embracing a data-driven culture is not just a solution—it is the key to transforming data into a powerful asset that propels AI effectiveness and drives business outcomes.
It starts with a solid data foundation.
Embrace AI now and position your organization as a leader in the digital age. Get in touch with us to learn how we can help you embark on your AI journey and capture transformative business value.
Contact Us